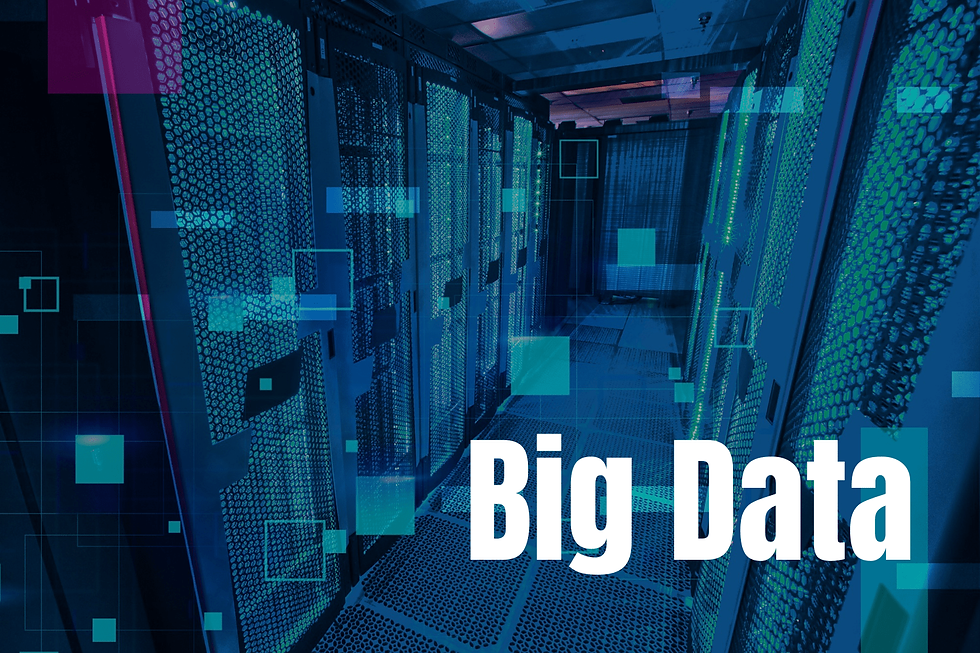
Big Data is a key component of audience measurement in the ever-changing media landscape, transforming the way content is watched and monetized. The definition of big data is examined in detail in this article, along with its importance and revolutionary effects on audience analytics.
Big Data, which includes enormous amounts of organized and unstructured information produced quickly from a variety of sources, is pervasive in the modern digital era. Gaining an understanding of the fundamentals of Big Data is essential to appreciating its application in audience measurement, where it offers detailed insights into the habits, tastes, and patterns of viewers.
The subtleties of big data, its use in the media sector, and its strategic benefits in terms of improving audience engagement and content strategy optimization will all be explained in detail in this extensive guide. Readers will acquire a thorough grasp of how Big Data supports audience measurement and stimulates innovation in media consumption through this investigation.
Understanding Big Data and Its Key Characteristics
Big Data refers to the vast and complex datasets generated in today's digital world, characterized by their immense size, speed of generation, and diverse formats. These datasets are beyond the capability of traditional data processing tools and require advanced analytics to extract meaningful insights. The concept of Big Data is best understood through its three fundamental characteristics: Volume, Velocity, and Variety.
Volume denotes the enormous amount of data produced every second. For instance, social media platforms generate terabytes of data daily from user interactions, posts, and multimedia content. This vast volume necessitates scalable storage solutions and sophisticated analytics tools to manage and interpret the data effectively.
Velocity refers to the speed at which data is generated and processed. In today's interconnected world, data flows continuously from various sources such as IoT devices, financial transactions, and online activities. For example, financial markets require real-time data analysis to make instantaneous trading decisions, highlighting the importance of high-velocity data processing.
Variety encompasses the diverse types of data generated from multiple sources. Big Data includes structured data from databases, unstructured data like text and images, and semi-structured data such as JSON files. An example is e-commerce platforms that analyze customer reviews (unstructured) alongside transactional data (structured) to enhance user experience.
Understanding these key characteristics of Big Data—Volume, Velocity, and Variety—enables organizations to harness its potential, driving innovation and informed decision-making across industries.
The Role of Audience Measurement in the Media Industry
Audience measurement is crucial for media companies and advertisers, providing vital insights into viewer demographics and behavior. By leveraging Big Data, media companies can analyze vast datasets to understand audience preferences and trends, which informs content creation and scheduling. This precise understanding of the audience enables media companies to tailor their offerings, enhancing viewer engagement and satisfaction.
For media buyers, accurate audience measurement is indispensable in optimizing advertising strategies. Understanding demographic composition—such as age, gender, income, and location—enables advertisers to target specific segments more effectively, ensuring that their campaigns reach the right audience. For example, an advertiser targeting young adults will prioritize platforms and time slots where this demographic is most active.
Moreover, demographic insights help media companies negotiate better ad rates. Advertisers are willing to pay a premium for access to highly targeted and engaged audiences, making accurate audience measurement a critical component of revenue generation.
Challenges and Opportunities in Leveraging Big Data for Audience Measurement
Leveraging Big Data for audience measurement presents both significant challenges and promising opportunities. Accurate audience measurement hinges on the effective use of Big Data technologies, yet several obstacles must be addressed to achieve this.
Key Challenges
One major challenge is data integration. Media companies must aggregate data from diverse sources such as social media, streaming platforms, and traditional TV. Ensuring that this data is harmonized and free from discrepancies is complex. Another challenge is real-time processing. With the high velocity of data generation, real-time analysis demands robust infrastructure and advanced analytics capabilities.
Privacy concerns also pose a significant challenge. As media companies collect vast amounts of personal data, they must navigate stringent data protection regulations to maintain audience trust. Finally, the sheer volume and variety of data require sophisticated storage solutions and analytical tools to extract meaningful insights efficiently.
Opportunities
Despite these challenges, the synergy between advanced technologies and robust processes offers substantial opportunities. Machine learning algorithms and AI can analyze large datasets more accurately and predict audience behavior patterns. Cloud computing provides scalable solutions for storing and processing vast amounts of data.
By leveraging these technologies, media companies can achieve more precise audience segmentation and deliver personalized content, enhancing viewer engagement. Additionally, effective utilization of Big Data enables advertisers to create targeted campaigns, maximizing ROI and improving customer experiences.
Big Data Processing Techniques for Audience Measurement
Big Data processing techniques are essential for effective audience measurement, enabling media companies to derive actionable insights from vast datasets. Two primary approaches, real-time processing and batch processing, each offer distinct advantages and challenges.
Real-Time Processing
Real-time processing involves the immediate analysis of data as it is generated. This technique is crucial for scenarios where timely insights are necessary, such as tracking live viewer engagement during a broadcast. Real-time processing uses technologies like Apache Kafka and Apache Flink to handle continuous data streams, allowing media companies to adjust content dynamically and optimize ad placements in real time.
Pros: Immediate insights, enhanced viewer engagement, and timely content adjustments.
Cons: High infrastructure costs, complex implementation, and potential data accuracy issues.
Batch Processing
Batch processing, on the other hand, involves the collection and analysis of large volumes of data at scheduled intervals. This method is suitable for comprehensive trend analysis and historical data comparisons. Tools like Apache Hadoop and Apache Spark are often used for batch processing, enabling the processing of massive datasets efficiently.
Pros: Cost-effective, handles large data volumes, suitable for in-depth analysis.
Cons: Delayed insights, less responsiveness to real-time events.
Both real-time and batch processing techniques are integral to Big Data processing for audience measurement. Real-time processing offers immediate feedback, crucial for dynamic content management, while batch processing provides robust analysis of historical trends. By strategically employing these techniques, media companies can enhance audience engagement and refine their content and advertising strategies.
Analyzing Big Data to Gain Audience Insights
Analyzing Big Data through various types of analytics—descriptive, predictive, and prescriptive—enables media companies to gain profound insights into audience behavior. Each analytic type offers unique benefits and applications in understanding and predicting audience dynamics.
Descriptive Analytics
Descriptive analytics involves summarizing historical data to identify patterns and trends. By utilizing techniques such as data aggregation and data mining, media companies can uncover audience viewing habits, peak engagement times, and content preferences. This type of analysis is fundamental for understanding past audience behavior and informing content strategies. For example, a streaming platform might use descriptive analytics to determine which genres are most popular during certain times of the year.
Predictive Analytics
Predictive analytics leverages statistical models and machine learning algorithms to forecast future audience behavior. This approach helps anticipate audience preferences and potential outcomes based on historical data. For instance, by analyzing past viewing patterns, predictive analytics can suggest which upcoming shows are likely to attract high viewership. This enables media companies to proactively tailor their content offerings and marketing efforts to meet anticipated demand.
Prescriptive Analytics
Prescriptive analytics goes a step further by providing actionable recommendations based on predictive insights. It uses optimization and simulation algorithms to suggest the best courses of action. In the context of audience measurement, prescriptive analytics can guide decision-making on content creation, scheduling, and advertising strategies, ensuring maximum engagement and revenue. For example, it can recommend the optimal release time for new content to maximize audience reach.
By integrating these analytics approaches, media companies can transform Big Data into valuable audience insights, driving strategic decisions and enhancing viewer satisfaction.
Evolution of Big Data Frameworks for Advanced Audience Measurement
The evolution of Big Data frameworks has significantly enhanced the capabilities for advanced audience measurement, catering to diverse data processing needs with increased efficiency and scalability. Key frameworks like Hadoop, Apache Spark, and Apache Kafka play pivotal roles in managing and analyzing massive datasets.
Hadoop
Hadoop revolutionized Big Data processing by enabling distributed processing of large data sets across clusters of computers. Its Hadoop Distributed File System (HDFS) and MapReduce programming model allow media companies to store and process vast amounts of audience data efficiently. This capability is crucial for batch processing tasks, such as analyzing historical viewing patterns and trends.
Apache Spark
Apache Spark offers substantial advantages for real-time audience measurement applications. Its in-memory processing capabilities provide significant speed improvements over Hadoop's disk-based processing. Spark's ability to handle both batch and stream processing makes it ideal for scenarios requiring immediate insights, such as tracking live audience engagement and optimizing content delivery in real time.
Apache Kafka
Apache Kafka is essential as a scalable, high-throughput messaging system for real-time data streaming. It facilitates the continuous collection and processing of audience data from various sources, ensuring that media companies can handle data with low latency. Kafka's robust architecture supports the seamless integration of real-time analytics, enabling timely and informed decision-making.
The integration of Hadoop, Apache Spark, and Apache Kafka within Big Data frameworks empowers media companies to conduct advanced audience measurement. These frameworks enable efficient data processing, real-time insights, and scalable data streaming, driving more informed strategies and enhancing viewer engagement.
Addressing the Complexities of Big Data Processing for Robust Audience Measurement
Effectively processing Big Data for audience measurement involves navigating several key challenges that demand advanced solutions and meticulous attention to detail. The inherent complexities of Big Data processing can impact the accuracy and reliability of audience insights.
Key Challenges
One of the primary challenges in Big Data processing is ensuring data quality and consistency at scale. Audience measurement data comes from diverse sources, including social media, streaming platforms, and traditional broadcast channels. Integrating this heterogeneous data requires rigorous validation and cleaning processes to eliminate discrepancies and maintain consistency.
Another significant challenge is managing the sheer volume and velocity of data. Real-time audience measurement necessitates the rapid processing of vast amounts of data, which can strain infrastructure and resources. Ensuring that the system can handle high-throughput data streams without latency or data loss is crucial for accurate and timely insights.
Scalability is also a critical concern. As audience data grows exponentially, the processing framework must scale seamlessly to accommodate increasing data loads. This requires robust architecture and advanced technologies, such as distributed computing and cloud-based solutions, to maintain performance and reliability.
Addressing these challenges in Big Data processing is essential for robust audience measurement. By ensuring data quality, managing volume and velocity, and implementing scalable solutions, media companies can derive accurate, actionable insights from their audience data, ultimately enhancing content strategies and viewer engagement.
The Future of Big Data in Shaping the Media Landscape
In the evolving media landscape, Big Data continues to be a cornerstone, driving significant changes in how audiences are measured and understood. Emerging technologies, particularly Artificial Intelligence (AI) and Machine Learning (ML), are at the forefront of this transformation, offering sophisticated tools to enhance audience measurement practices.
AI and ML technologies harness Big Data to deliver granular insights into audience behavior, preferences, and engagement. These advanced analytics go beyond traditional metrics, enabling media companies to predict trends and tailor content with unprecedented precision. Machine learning algorithms can analyze vast datasets in real-time, identifying patterns and correlations that would be impossible for human analysts to discern. This capability allows for more accurate targeting and personalization, enhancing the overall user experience.
Moreover, Big Data facilitated by AI and ML is revolutionizing advertising strategies. By understanding viewer habits and preferences, media companies can optimize ad placements, improving ROI for advertisers. Predictive analytics derived from Big Data enable dynamic content delivery, ensuring that the right content reaches the right audience at the right time.
The integration of AI and ML in audience measurement signifies a shift towards more intelligent and responsive media strategies. As these technologies continue to evolve, the potential for Big Data to reshape the media landscape becomes increasingly apparent, promising more refined and effective audience engagement methodologies.
Conclusion
In conclusion, leveraging the power of Big Data is crucial for businesses aiming to enhance their audience measurement strategies. By integrating advanced technologies like Artificial Intelligence and Machine Learning, companies can gain deeper insights into audience behaviors and preferences.
This strategic use of Big Data enables more precise targeting, personalized content delivery, and optimized advertising efforts. As the media landscape continues to evolve, embracing Big Data will be key to staying competitive and effectively engaging with audiences. Businesses should harness these advancements to drive their growth and success in an increasingly data-driven world.
Comentarios